High-quality data can enable companies to remain competitive and invincible in times of economic turmoil. With universal in-depth data quality, enterprises can trust all data that meets all needs at any time. A strategic and systematic approach can help enterprises correctly study their data quality projects. What are the important features of data and how to do data quality management?
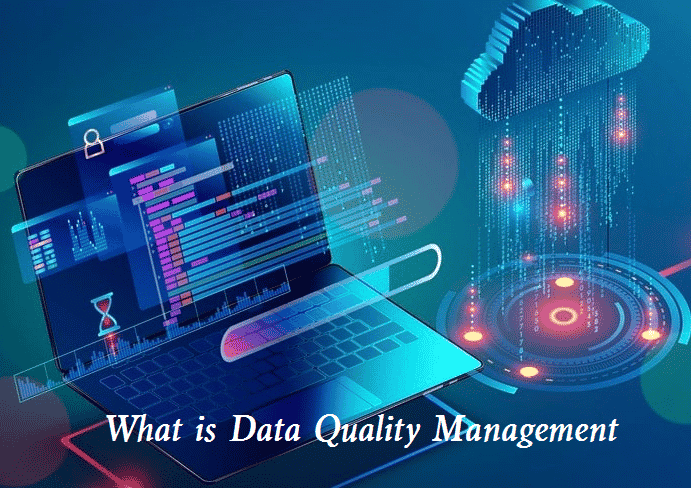
What is Data Quality Management?
Data quality management refers to a series of management activities such as identification, measurement, monitoring, and early warning of various data quality problems that may arise in each stage of the life cycle of data planning, acquisition, storage, sharing, maintenance, application, and extinction, and further improve the data quality by improving and improving the management level of the organization. Data quality management is a process of circular management, and its ultimate goal is to enhance the value of data in use through reliable data.
6 Characteristics of Data Quality
Integrity
Integrity refers to whether the data records and information are complete and whether there is any missing. Data loss mainly includes the loss of records and the loss of information about a field in records. Both of them will cause inaccurate statistical results. Therefore, integrity is the most basic guarantee for data quality. Two aspects need to be considered in monitoring: whether the number of data pieces is less, and whether the values of some fields are missing integrity monitoring, which mostly occurs in log level monitoring. Generally, data integrity verification is performed during data access.
Accuracy
Accuracy refers to whether the information and data recorded in the data are accurate and whether there is abnormal or wrong information. Intuitively speaking, it depends on whether the data is accurate. Generally, the monitoring of accuracy focuses on the monitoring of business result data, such as whether the daily activity, revenue, and other data are normal.
Consistency
Consistency refers to whether the results of the same indicator are consistent in different places. Data inconsistency often occurs after the data system reaches a certain complexity, and the same indicator will be calculated in multiple places. Due to different calculation caliber or developers, it is easy to cause different results of the same indicator.
Timeliness
Timeliness after ensuring the integrity, accuracy and consistency of the data, the next step is to ensure that the data can be produced in time, so as to reflect the value of the data. Timeliness is easy to understand. It mainly refers to whether the data is calculated fast enough. This can be reflected in the data quality monitoring whether the monitoring result data is calculated before the specified time point.
Normative
Whether normative data is stored according to the required rules has certain semantic significance. Such as certificate verification, mailbox verification, IP address verification, phone format verification, zip code format verification, date format verification, null value or empty string verification, and numeric format verification.
Uniqueness
Whether the unique data is duplicated. For example, the unique value of a field, the duplicate value of a field, and so on.
How to Do Data Quality Management
- Probe data content, structure, and exceptions
The first step is to probe the data to find and evaluate the content, structure, and exceptions of the data. Through exploration, the advantages and disadvantages of data can be identified to help enterprises determine project plans. A key goal is to clearly identify data errors and problems, such as inconsistencies and redundancies that will pose a threat to business processes. - Establish data quality metrics and define objectives
Business personnel and its personnel provide a common platform to establish and improve metrics. Users can track the compliance of metrics in the data quality scorecard. - Design and implement data quality business rules
Define the data quality rules of the enterprise, that is, reusable business logic, and manage how to clean data and parse fields and data used to support target applications. Businesses and their departments use role-based functions to design, test, improve and implement data quality business rules together to achieve the best results. - Build data quality rules into the data integration process
Data quality services consist of centrally managed, application-independent, and reusable business rules that can be used to perform exploration, cleaning, standardization, name and address matching, and monitoring. - Check for exceptions and improve rules
After implementing the data quality process, most records will be cleaned and standardized, and the data quality objectives set by the enterprise will be achieved. However, it is inevitable that there will still be some inferior data that has not been cleaned. At this time, it is necessary to improve the business rules to control the data quality. - Monitor data quality against objectives
Data quality control should not be a one-time activity. Relative goals and continuous monitoring and management of data quality throughout business applications are critical to maintaining and improving high levels of data quality performance.